Séminaire de mathématiques actuarielles et financières: «Quasi-Monte Carlo for multivariate distributions via generative neural networks»
Conférencier: Marius Hofert, University of Waterloo
Résumé / Abstract : Generative moment matching networks are introduced as quasi-random number generators (QRNGs) for copula models in order to estimate expectations with variance reduction. So far, QRNGs for copula models required a careful design, exploiting specific properties (such as conditional distributions) of the target copula or the underlying quasi-Monte Carlo (QMC) point set, and were only tractable for few models. Utilizing generative moment matching networks allows one to construct QRNGs for a much larger variety of multivariate distributions without such restrictions. Once trained with a pseudo-random sample, these neural networks only require a multivariate standard uniform randomized QMC point set as input and are thus fast in estimating expectations of interest under dependence. Numerical examples are considered to demonstrate the approach.
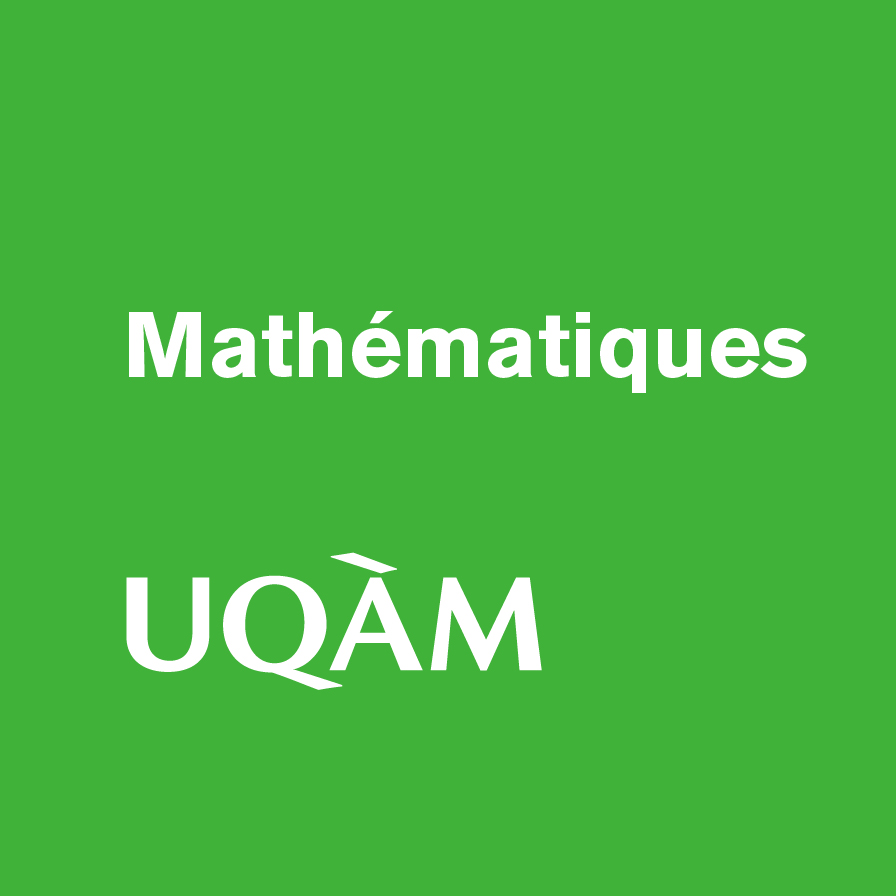
Date / heure
Lieu
Montréal (QC)